Buoyant skies linger overhead,
bulging at the seams
with surging intent;
capricious threats
that fall indiscriminately
against the statistical fortitude
of our modelled routines.
Searching for clarity
we train machines
to find patterns
amongst the cyclical nature
of extreme behaviours.
Cutting through complexities,
algorithmic digits point nervously
to the faultless correlation
with our own excess.
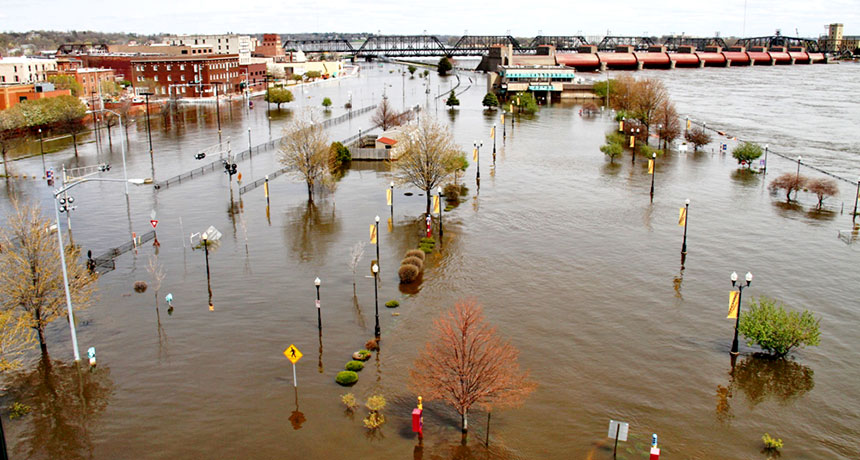
This poem is inspired by recent research, which has used machine learning to understand why extreme precipitation days in the Midwestern United States are becoming more frequent.
Climate change is known to have increased the frequency and intensity of extreme precipitation in recent years. For example, global warming has driven heavier rain and snowfall by creating a warmer atmosphere that can hold more moisture. However, revealing the specific impacts that climate change will have on extreme weather events is difficult, especially at the regional level, where global climate models do not necessarily have the spatial resolution required. Machine learning (which can be thought of as computer algorithms that improve automatically through experience) offer one potential solution to this problem, due to their ability to learn complex, non-linear patterns at high resolution.
In this new study, researchers trained a machine learning algorithm to identify atmospheric circulation patterns associated with extreme precipitation over a specific region in the United States: the Upper Mississippi Watershed and the eastern portion of the Missouri Watershed. This is a highly flood-prone region in the U.S., which spans parts of nine states, and which has seen extreme precipitation and major flooding become more frequent in recent decades. The algorithm was used to correctly identify over 90% of the extreme precipitation days in this region from 1981 to 2019, performing better than other more traditional statistical methods that were also tested by the researchers. In successfully predicting these events, the algorithm also revealed that multiple factors are responsible for the recent increase in Midwest extreme precipitation. For example, since the turn of the century, the atmospheric pressure patterns that lead to extreme Midwest precipitation have become more frequent, increasing at a rate of about one additional day per year. While this research has initially focussed on understanding extreme precipitation in the Midwest, it also demonstrates how machine learning might be applied to understand changes in extreme weather events in other regions across the world.
Discover more from The Poetry of Science
Subscribe to get the latest posts sent to your email.
Bravo! One of your best.
Now society has to figure out how to deal with “the cyclical nature
of (our) extreme behaviours.”
Thank you! And yes, absolutely. It seems we are missing the most obvious of variables… 🙁
In addition to global weirdness, DoD has been experimenting with local weather modification for decades. I wouldn’t be surprised if some of the unprecedented weather blips recently are man made.
I kept coming back to :
Searching for clarity
we train machines
to find patterns
There’s sonorous simplicity here among a field of flowery alliteration that hints at a greater truth with “faultless correlation,” but an extra day of excessive weather every year is terrifying. Well done poem, however.
Thanks James. 😀